Uncrewed air traffic
Allowing individual unmanned aerial vehicles (UAVs) to adjust their trajectory to avoid collisions is an effective way to safely manage high traffic flows without the need for central scheduling. Measures such as dividing a stream of UAVs into two or forming tightly bunched UAV platoons could further increase traffic flow and be used in future air traffic management of commercial UAVs.
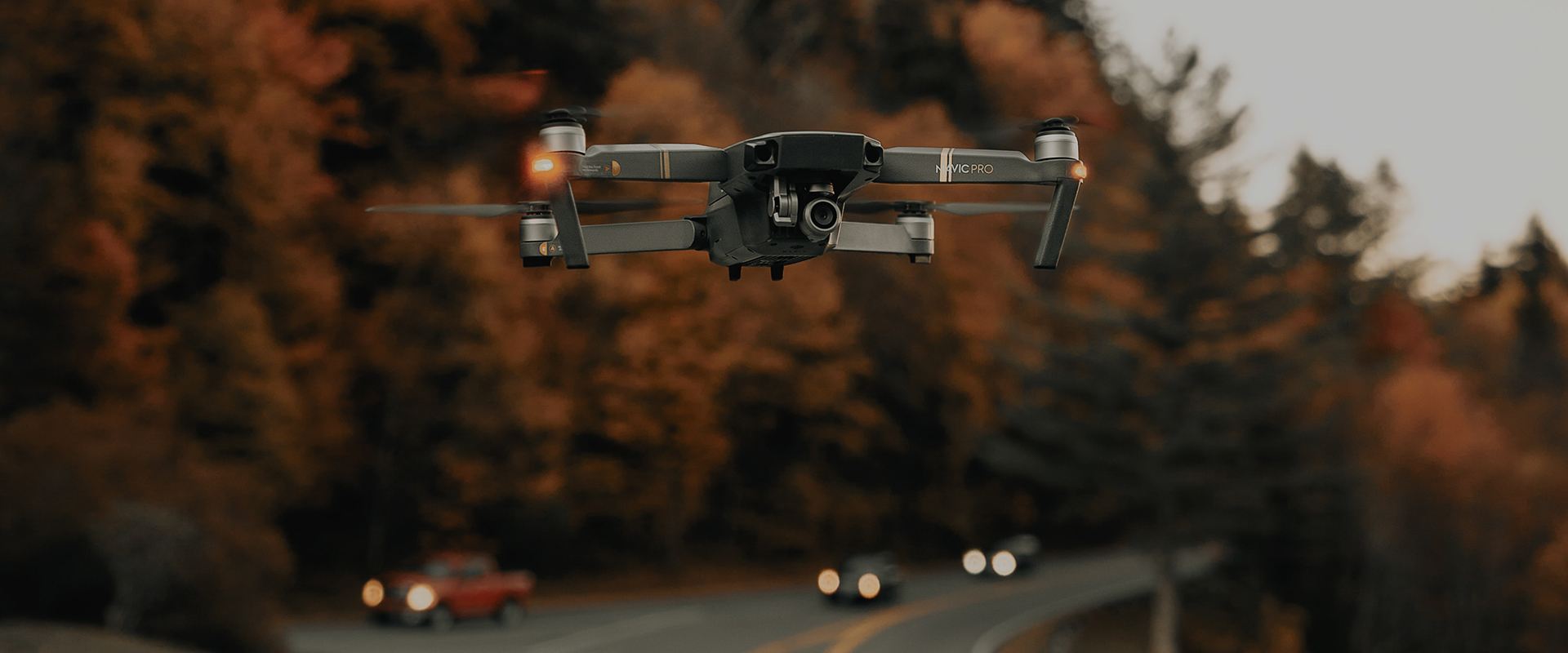
PhD student: William Bonnell
Supervisory team: Eddie Wilson, David Harvey and Angus Johnson
UAV superhighways
From infrastructure inspection to search-and-rescue operations, unmanned aerial vehicles (UAVs) have started to serve as multi-purpose tools and platforms. The next potential development? Fleets of UAVs being used for delivery services, unimpeded by road traffic.
The skies don’t offer unlimited capacity for UAVs though. UAVs must operate in a narrow layer of airspace, which means they are likely to interact where delivery routes cross. Traditional air traffic is currently managed through centrally controlled scheduling, but this will be inappropriate for handling the high numbers of UAVs for logistics services. A different approach is required.
We have investigated whether higher traffic densities could be supported by UAVs managing interactions themselves using local observations.
UAVs at a crossroads
The most critical UAV interaction to resolve for managing traffic is where UAV paths cross.
We simulated junctions with two streams of UAVs arriving from perpendicular directions, such as might arise with a series of UAVs passing between logistics hubs.
UAVs were allowed to steer away from a potential collision by rotating their trajectory to their right until the collision was no longer predicted, and then continue directly towards its destination. This simple approach allowed UAVs to self-manage potential collisions without changing speed.
Streams of UAVs were able to cross each other’s path without collisions up to a maximum rate of traffic flow that a perfect scheduling system could allow. Each UAV followed a unique path, depending on the local situation it encountered, meaning crossing dynamics could be highly complex.
Smart management of UAV traffic
The delay experienced by UAVs navigating the crossing was highly sensitive to traffic rate and increased nonlinearly at high flows of UAVs.
We exploited this sensitivity by dividing one of the UAV streams into two prior to a crossing to create two junctions with the other UAV stream. This more than halved the overall crossing delays for the same overall flow of UAVs compared to a single junction approach.
This simple splitting process could be continued in the future to create multiple crossing points for even higher traffic flows.
We also found that forming platoons of small groups of tightly spaced UAVs created larger gaps that UAVs in the other stream could navigate more easily. This simple measure increased the delay of some individual UAVs as they waited to form a platoon but reduced the mean delay for all UAVs by up to 20%.
Real-world UAV
Our research has shown how UAV traffic management can be improved but have assumed perfect sensing and communication of UAV position, direction, and speed. Real-world limitations on communications and safety margins might mean a lower practical limit of traffic flow than those shown here.
Nonetheless, management of individual UAV motion based on local observations shows great promise to avoid collisions at crossings under high traffic loads, which could unlock new opportunities for UAV development.